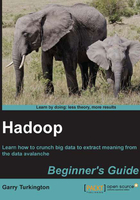
Time for action – WordCount, the Hello World of MapReduce
Many applications, over time, acquire a canonical example that no beginner's guide should be without. For Hadoop, this is WordCount – an example bundled with Hadoop that counts the frequency of words in an input text file.
- First execute the following commands:
$ hadoop dfs -mkdir data $ hadoop dfs -cp test.txt data $ hadoop dfs -ls data Found 1 items -rw-r--r-- 1 hadoop supergroup 16 2012-10-26 23:20 /user/hadoop/data/test.txt
- Now execute these commands:
$ Hadoop Hadoop/hadoop-examples-1.0.4.jar wordcount data out 12/10/26 23:22:49 INFO input.FileInputFormat: Total input paths to process : 1 12/10/26 23:22:50 INFO mapred.JobClient: Running job: job_201210262315_0002 12/10/26 23:22:51 INFO mapred.JobClient: map 0% reduce 0% 12/10/26 23:23:03 INFO mapred.JobClient: map 100% reduce 0% 12/10/26 23:23:15 INFO mapred.JobClient: map 100% reduce 100% 12/10/26 23:23:17 INFO mapred.JobClient: Job complete: job_201210262315_0002 12/10/26 23:23:17 INFO mapred.JobClient: Counters: 17 12/10/26 23:23:17 INFO mapred.JobClient: Job Counters 12/10/26 23:23:17 INFO mapred.JobClient: Launched reduce tasks=1 12/10/26 23:23:17 INFO mapred.JobClient: Launched map tasks=1 12/10/26 23:23:17 INFO mapred.JobClient: Data-local map tasks=1 12/10/26 23:23:17 INFO mapred.JobClient: FileSystemCounters 12/10/26 23:23:17 INFO mapred.JobClient: FILE_BYTES_READ=46 12/10/26 23:23:17 INFO mapred.JobClient: HDFS_BYTES_READ=16 12/10/26 23:23:17 INFO mapred.JobClient: FILE_BYTES_WRITTEN=124 12/10/26 23:23:17 INFO mapred.JobClient: HDFS_BYTES_WRITTEN=24 12/10/26 23:23:17 INFO mapred.JobClient: Map-Reduce Framework 12/10/26 23:23:17 INFO mapred.JobClient: Reduce input groups=4 12/10/26 23:23:17 INFO mapred.JobClient: Combine output records=4 12/10/26 23:23:17 INFO mapred.JobClient: Map input records=1 12/10/26 23:23:17 INFO mapred.JobClient: Reduce shuffle bytes=46 12/10/26 23:23:17 INFO mapred.JobClient: Reduce output records=4 12/10/26 23:23:17 INFO mapred.JobClient: Spilled Records=8 12/10/26 23:23:17 INFO mapred.JobClient: Map output bytes=32 12/10/26 23:23:17 INFO mapred.JobClient: Combine input records=4 12/10/26 23:23:17 INFO mapred.JobClient: Map output records=4 12/10/26 23:23:17 INFO mapred.JobClient: Reduce input records=4
- Execute the following command:
$ hadoop fs -ls out Found 2 items drwxr-xr-x - hadoop supergroup 0 2012-10-26 23:22 /user/hadoop/out/_logs -rw-r--r-- 1 hadoop supergroup 24 2012-10-26 23:23 /user/hadoop/out/part-r-00000
- Now execute this command:
$ hadoop fs -cat out/part-0-00000 This 1 a 1 is 1 test. 1
What just happened?
We did three things here, as follows:
- Moved the previously created text file into a new directory on HDFS
- Ran the example WordCount job specifying this new directory and a non-existent output directory as arguments
- Used the
fs
utility to examine the output of the MapReduce job
As we said earlier, the pseudo-distributed mode has more Java processes, so it may seem curious that the job output is significantly shorter than for the standalone Pi. The reason is that the local standalone mode prints information about each individual task execution to the screen, whereas in the other modes this information is written only to logfiles on the running hosts.
The output directory is created by Hadoop itself and the actual result files follow the part-nnnnn convention illustrated here; though given our setup, there is only one result file. We use the fs -cat
command to examine the file, and the results are as expected.
Note
If you specify an existing directory as the output source for a Hadoop job, it will fail to run and will throw an exception complaining of an already existing directory. If you want Hadoop to store the output to a directory, it must not exist. Treat this as a safety mechanism that stops Hadoop from writing over previous valuable job runs and something you will forget to ascertain frequently. If you are confident, you can override this behavior, as we will see later.
The Pi and WordCount programs are only some of the examples that ship with Hadoop. Here is how to get a list of them all. See if you can figure some of them out.
$ hadoop jar hadoop/hadoop-examples-1.0.4.jar
Have a go hero – WordCount on a larger body of text
Running a complex framework like Hadoop utilizing five discrete Java processes to count the words in a single-line text file is not terribly impressive. The power comes from the fact that we can use exactly the same program to run WordCount on a larger file, or even a massive corpus of text spread across a multinode Hadoop cluster. If we had such a setup, we would execute exactly the same commands as we just did by running the program and simply specifying the location of the directories for the source and output data.
Find a large online text file—Project Gutenberg at http://www.gutenberg.org is a good starting point—and run WordCount on it by copying it onto the HDFS and executing the WordCount example. The output may not be as you expect because, in a large body of text, issues of dirty data, punctuation, and formatting will need to be addressed. Think about how WordCount could be improved; we'll study how to expand it into a more complex processing chain in the next chapter.
Monitoring Hadoop from the browser
So far, we have been relying on command-line tools and direct command output to see what our system is doing. Hadoop provides two web interfaces that you should become familiar with, one for HDFS and the other for MapReduce. Both are useful in pseudo-distributed mode and are critical tools when you have a fully distributed setup.
Point your web browser to port 50030 on the host running Hadoop. By default, the web interface should be available from both the local host and any other machine that has network access. Here is an example screenshot:

There is a lot going on here, but the immediately critical data tells us the number of nodes in the cluster, the filesystem size, used space, and links to drill down for more info and even browse the filesystem.
Spend a little time playing with this interface; it needs to become familiar. With a multinode cluster, the information about live and dead nodes plus the detailed information on their status history will be critical to debugging cluster problems.
The JobTracker UI is available on port 50070 by default, and the same access rules stated earlier apply. Here is an example screenshot:

This is more complex than the HDFS interface! Along with a similar count of the number of live/dead nodes, there is a history of the number of jobs executed since startup and a breakdown of their individual task counts.
The list of executing and historical jobs is a doorway to much more information; for every job, we can access the history of every task attempt on every node and access logs for detailed information. We now expose one of the most painful parts of working with any distributed system: debugging. It can be really hard.
Imagine you have a cluster of 100 machines trying to process a massive data set where the full job requires each host to execute hundreds of map and reduce tasks. If the job starts running very slowly or explicitly fails, it is not always obvious where the problem lies. Looking at the MapReduce web UI will likely be the first port of call because it provides such a rich starting point to investigate the health of running and historical jobs.