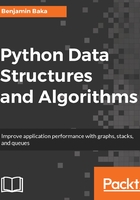
Arrays
The array module defines a datatype array that is similar to the list datatype except for the constraint that their contents must be of a single type of the underlying representation, as is determined by the machine architecture or underlying C implementation.
The type of an array is determined at creation time and it is indicated by one of the following type codes:

The array objects support the following attributes and methods:

Array objects support all the normal sequence operations such as indexing, slicing, concatenation, and multiplication.
Using arrays, as opposed to lists, is a much more efficient way of storing data that is all of the same type. In the following example, we have created an integer array of the digits from 0 to 1 million minus 1, and an identical list. Storing 1 million integers in an integer array requires around 45% of the memory of an equivalent list:

Because we are interested in saving space, that is, we are dealing with large datasets and limited memory size, we usually perform in-place operations on arrays, and only create copies when we need to. Typically, enumerate is used to perform an operation on each element. In the following snippet, we perform the simple operation of adding one to each item in the array:

It should be noted that when performing operations on arrays that create lists, such as list comprehensions, the memory efficiency gains of using an array in the first place will be negated. When we need to create a new data object, a solution is to use a generator expression to perform the operation, for example:

Arrays created with this module are unsuitable for work that requires a matrix of vector operations. In the next chapter, we will build our own abstract data type to deal with these operations. Also important for numerical work is the NumPy extension, available at www.numpy.org .